Table.AddKey()可以抽象地概括为function(table as table, columns as list, isPrimary as logical) as table,意思为该函数的第一个参数为表格,第二个参数为组成第一个参数的键的一个或者多个字段,这些字段需要以文本的形式作为传入第二个参数的串列的元素,最后一个参数为逻辑型变量用来指定键的种类: true代表主键而false代表非主键。
经过一番探索,发现在一对多的模型中,对一边(One Side)的表格使用Table.AddKey()后能够在数据比较多的情况下大幅度减少左表为一边的连接所需要的时间。要得到这个结论,首先需要在Excel工作表中建立一个参数表(DataSize):
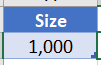
接着,在Power Query中定义以下表格(Product),该表格的第一列为1到26的阿拉伯数字,第二列为对应的26个英文字母:
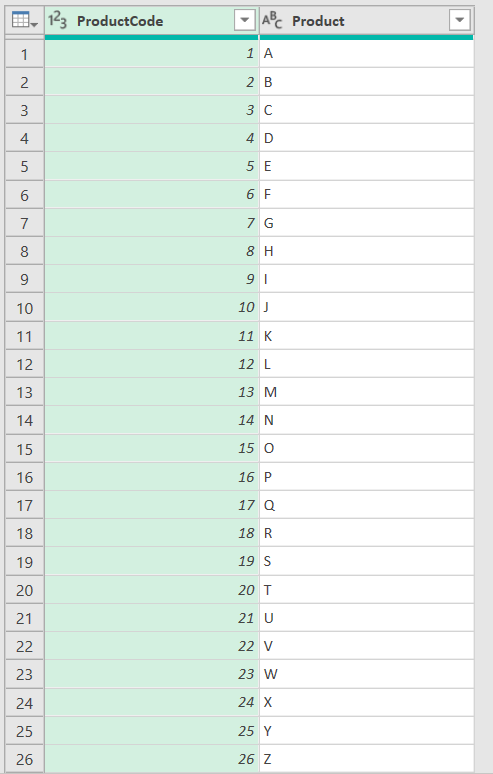
对以上表格使用= Table.AddKey( Product, { "ProductCode" }, true )生成的ProductwithKey表格,从外观上来看与原表并无区别:
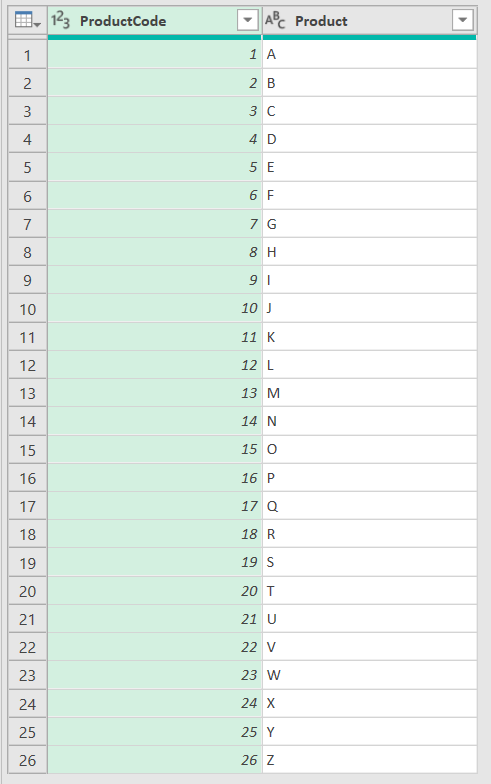
然后在Power Query里构造行数随着DataSize变化的Sales表格,这个表格的第一列只含有1到26的整数,第二列为从1开始公差为1的等差数列:
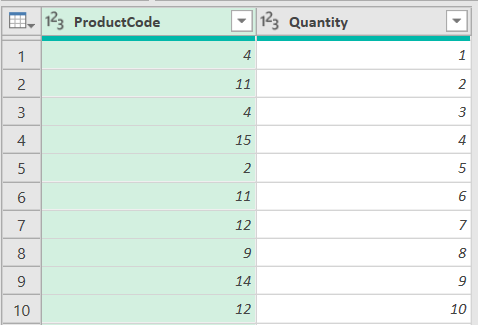
在Query查询中,内连接的左表为Product而右表为Sales:
let Source= Table.NestedJoin( Product, {"ProductCode"}, Sales, {"ProductCode"}, "NTable", JoinKind.Inner ), Aggregation= Table.AggregateTableColumn( Source, "NTable", { { "Quantity", List.Sum, "Total", Int64.Type } } ), RemoveCol= Table.SelectColumns( Aggregation, { "Product", "Total" } ) in RemoveCol
在QuerywithKey查询中,内连接的左表为ProductwithKey而右表为Sales:
let Source= Table.NestedJoin( ProductwithKey, {"ProductCode"}, Sales, {"ProductCode"}, "NTable", JoinKind.Inner ), Aggregation= Table.AggregateTableColumn( Source, "NTable", { { "Quantity", List.Sum, "Total", Int64.Type } } ), RemoveCol= Table.SelectColumns( Aggregation, { "Product", "Total" } ) in RemoveCol
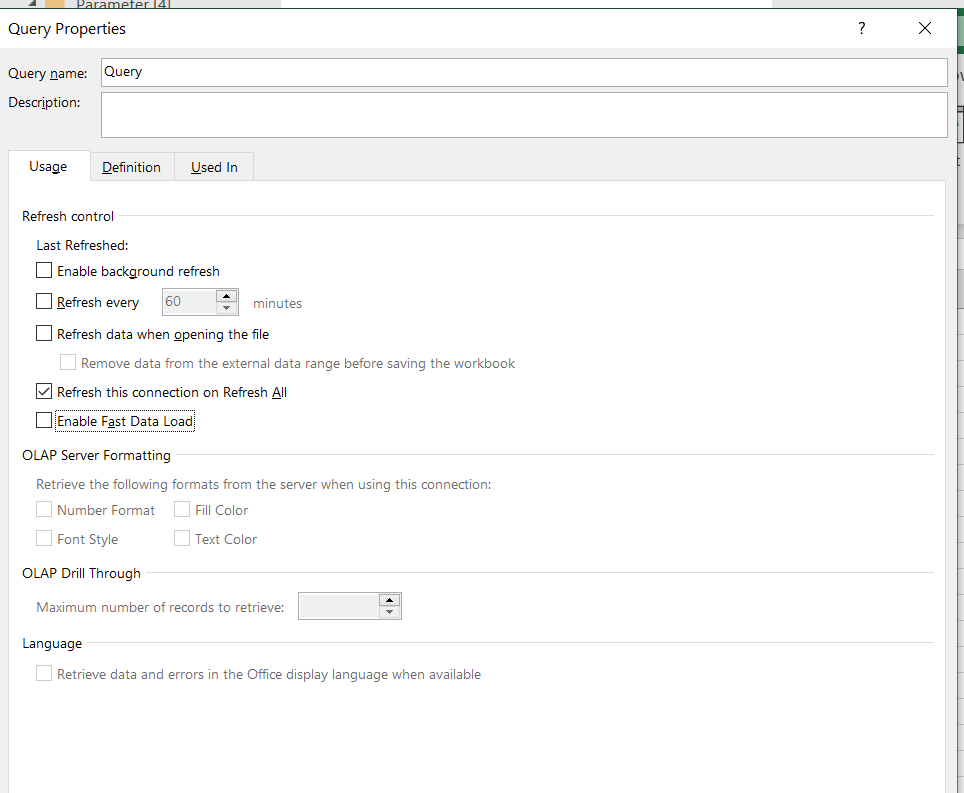
接下来分别取消Query与QuerywithKey的背景更新,之后运行宏Efficiency:
Sub Efficiency() Dim StartTime As Single Dim EndTime1 As Single Dim EndTime2 As Single With Application .Calculation = xlCalculationManual .ScreenUpdating = False End With StartTime = Timer With ThisWorkbook .Connections("Query - QueryWithKey").Refresh EndTime1 = Timer .Connections("Query - Query").Refresh EndTime2 = Timer End With MsgBox Format((EndTime1 - StartTime) / (EndTime2 - EndTime1), "#,##0.00%") With Application .Calculation = xlCalculationAutomatic .ScreenUpdating = False End With End Sub
在宏Efficiency中EndTime1 - StartTime为QueryWithKey更新所需要的时间,EndTime2 - EndTime1为Query更新所消耗的时间,两者之比的大小反映了Table.AddKey()对查询所需要的时间的影响,大于1说明加了键后更新所需要的时间更多,小于1说明加了键后代码得到优化。以下是测试的结果:
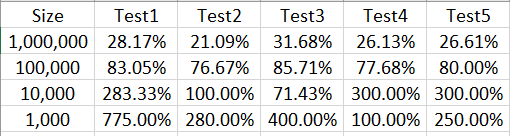
以上表格说明行数介于100,000与1,000,000之间时,在使用Table.AddKey()后代码的运行时间显著减少,但是数据的行数在1,000于10,000之间时代码运行的时间在多数测试中反而是增加的。